Research Statement
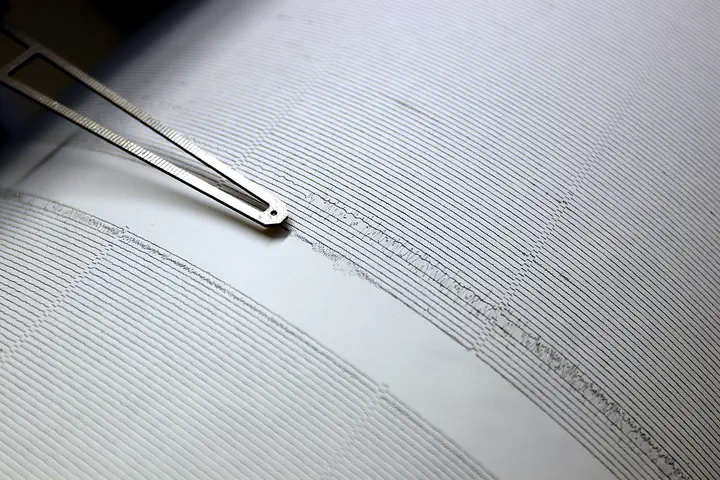
Geophysics is largely an observational science, and the ability to interpret measured data is fundamental to progress.
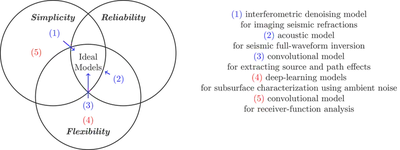
My research seeks simple, reliable, and flexible signal models for seismic inversion that improve over their predecessors.
It allows for an accurate characterization of the subsurface structures, at both global and exploration scales, and also of natural and induced seismicity. Our current models are established on well-understood physical principles. However, they have to be allowed to evolve with the developments in instrumentation and data availability. For example, conventional signal models might not be optimal for processing waves that are recorded using fiber optic cables. More importantly, my research will leverage vast amounts of data that are being generated by our seismometers to show that improved models can also be learned directly from the data. Researchers in disciplines such as computer science and engineering are being constantly challenged to develop methods that learn models given big data. However, those methods don’t constitute a skeleton that can build on the top of the existing physical principles. Internalizing their methods into seismic imaging has to be done in a way that we don’t ignore the physics that is at the core of our systems. My role in the geophysics department is to internalize advances in disciplines like electrical engineering, applied mathematics and computer science.
For a model to succeed in its mission of treating the data, it must be a good compromise between three qualities: simplicity, reliability, and flexibility. There virtues in the view of the experimental goal and setup. Inverse problems based on simple models are stable and easy to solve; although, it is questionable if these models are appropriate for complex measurements. Reliable models capture the true essence of the data; however, they may not necessarily explain all the details in the data. Flexible models have the capacity to explain the data to the fullest, however, given insufficient data, they fail to provide reliable estimates; they should often be modified with additional constraints to have a reliable inversion. I have depicted the seismic inversion models that I developed in the past in the Figure. In this figure, the models are torn between the three qualities, where my contribution allowed development of ideal models.