Geophysical Inversion
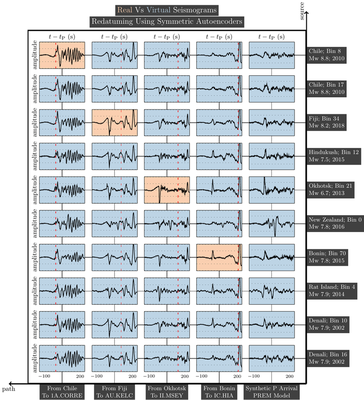
Can you spot real seismograms in the figure above? Here, the time series highlighted in orange are real and the rest are virtual: similar to deepfakes in computer vision, we generated virtual seismograms after training a symmetry-constrained autoencoder neural network. Why should we care about the virtual seismograms? It can be observed that the earthquake source features are clearly interpretable in the right-most column with virtual seismograms. Otherwise, the earthquake source information is typically obfuscated in the real seismograms because of the wave scattering (roughly indicated by the dashed red line) in the subsurface. In this figure, it can be noticed that the effects of scattering are systematically minimized from left to right facilitating accurate characterization of several complex earthquake sources.
Seismology offers a means to investigate the Earth’s interior to the greatest depth, with higher resolution and accuracy than any other branch of geophysics. To improve the quantitative picture of seismology, our group is currently developing innovative physics-based deep-learning frameworks. These methods can process large-$N$ and large-$t$ waveforms effectively. Additionally, they can isolate signals related to a physical phenomenon of interest from the remaining coupled nuisance effects that obfuscate measurements in all branches of geophysics. Some challenging problems that we are aiming to solve by designing neural networks include:
- isolation of earthquake-related information from seismograms to better constrain kinematic rupture models;
- decomposition of multicomponent seismograms into individual phases (P and S) for better target-oriented imaging and interpretation;
- reliable extraction of time-lapse signatures from passive seismic data to assist monitoring of carbon storage and sequestration.
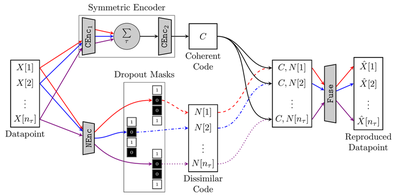